Recommender System from Scratch
Collaborative Filtering · Content-Based Methods · Hybrid Recommendation
Overview
This project demonstrates foundational and intermediate-level recommender system models built entirely from scratch, covering popularity-based, regression-based, collaborative filtering, and content-based recommendation approaches.
The project is divided into three stages:
- Chapter 1: Foundations Data exploration, simple popularity models, and regression-based rating prediction.
- Chapter 2: Collaborative Filtering User-user and item-item collaborative filtering methods with KNN and similarity-based predictions.
- Chapter 3: Content-Based Recommendation Building feature representations of items using text preprocessing and TF-IDF, user profile construction, personalized content-based recommendations, and precision/recall evaluation.
Each model is implemented and evaluated step-by-step to build a comprehensive understanding of recommendation system techniques.
Technology Stack
- Programming Language:
Python - Data Analysis and Manipulation:
pandas, numpy - Machine Learning and Evaluation:
scikit-learn - Text Processing:
NLTK - Visualization:
matplotlib, seaborn
Dataset Description
- Joke Ratings Dataset:
A user-item matrix where users rate jokes from 1 to 100; used for collaborative filtering and content-based methods. - Joke Text Dataset:
Raw textual content of jokes, preprocessed to generate TF-IDF item features. - Stopword List:
Used for cleaning joke texts during feature engineering.
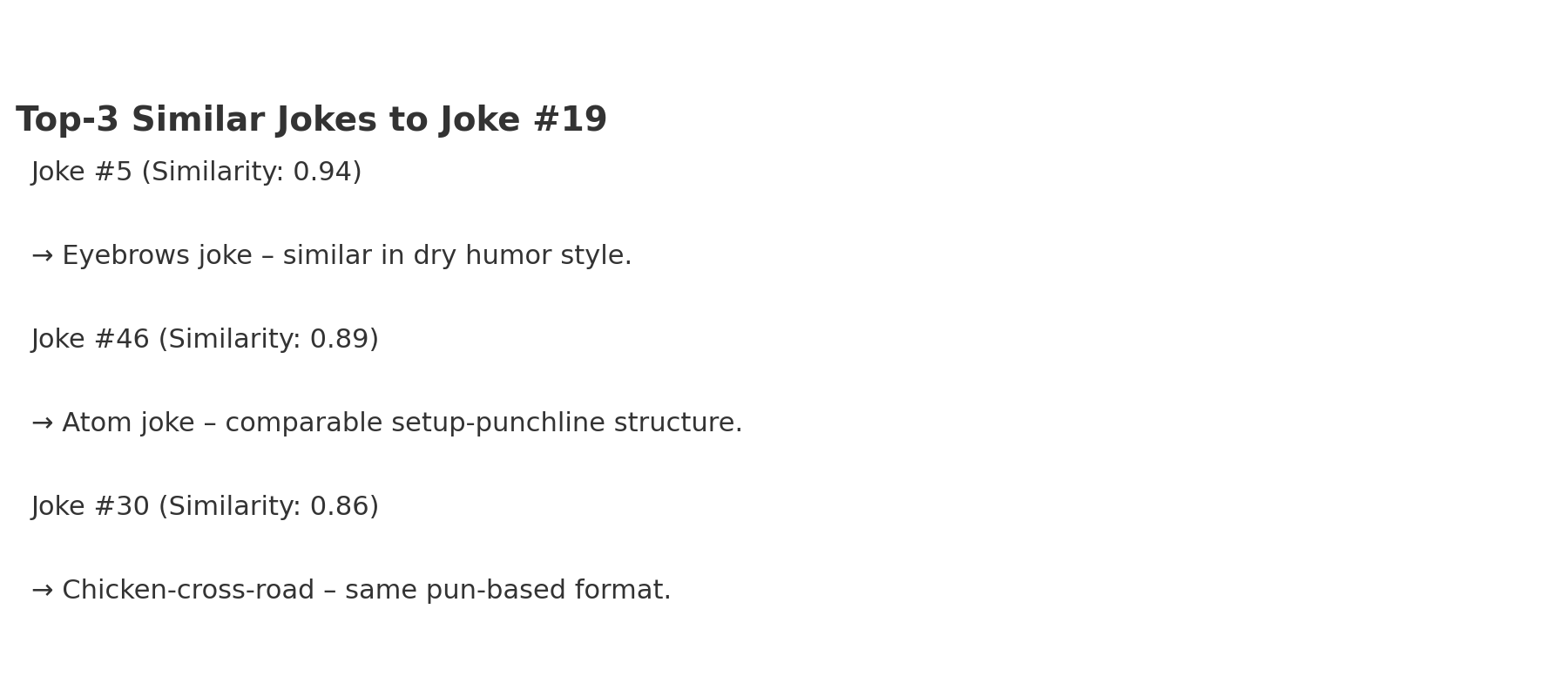
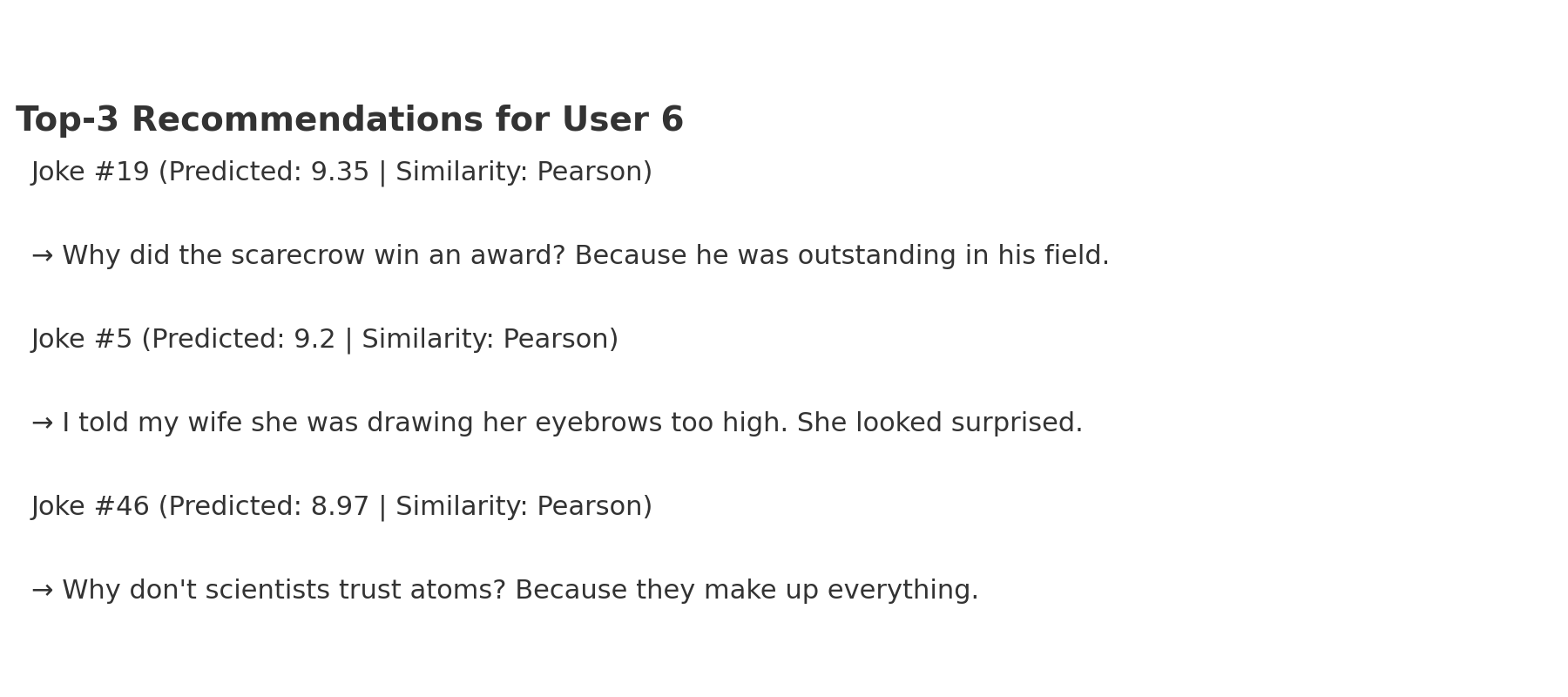
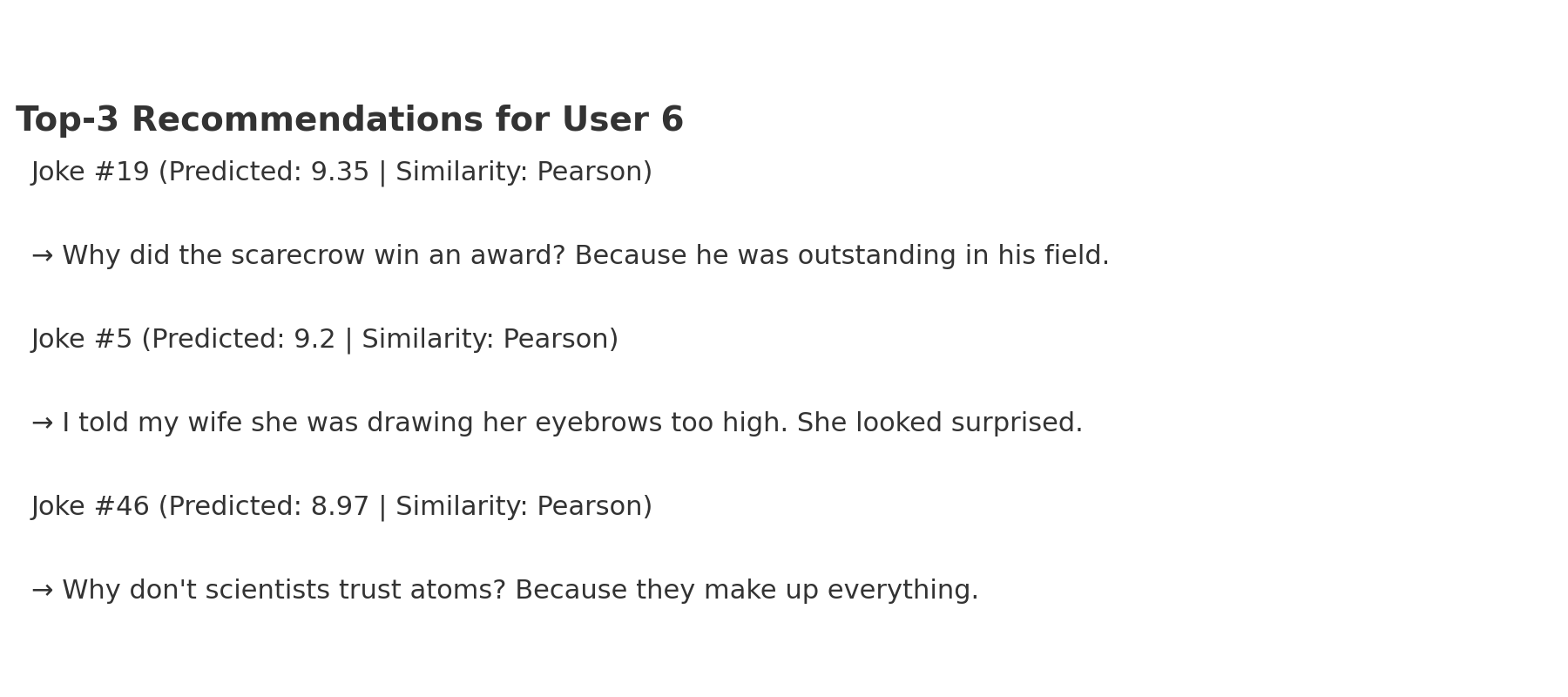
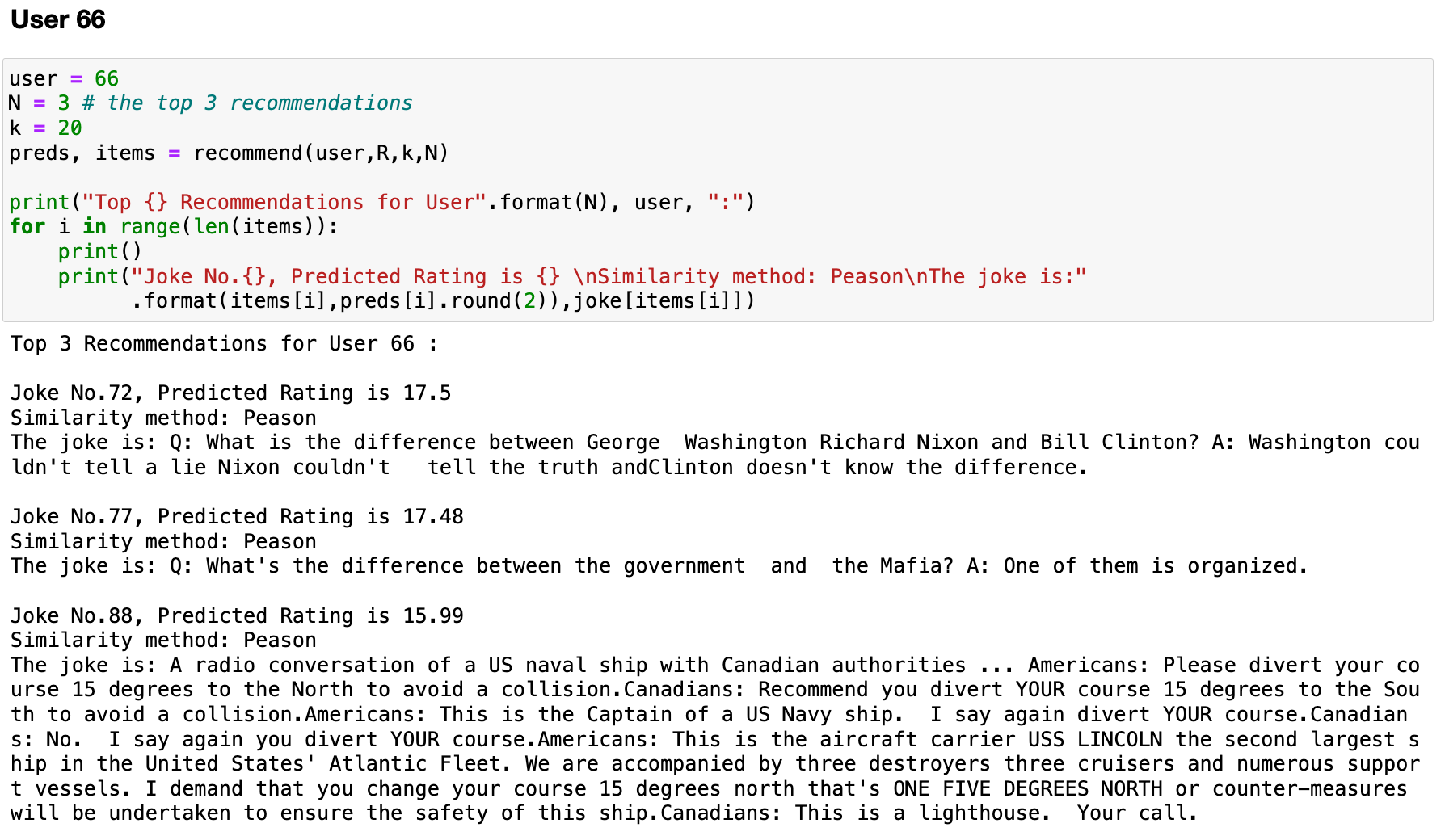
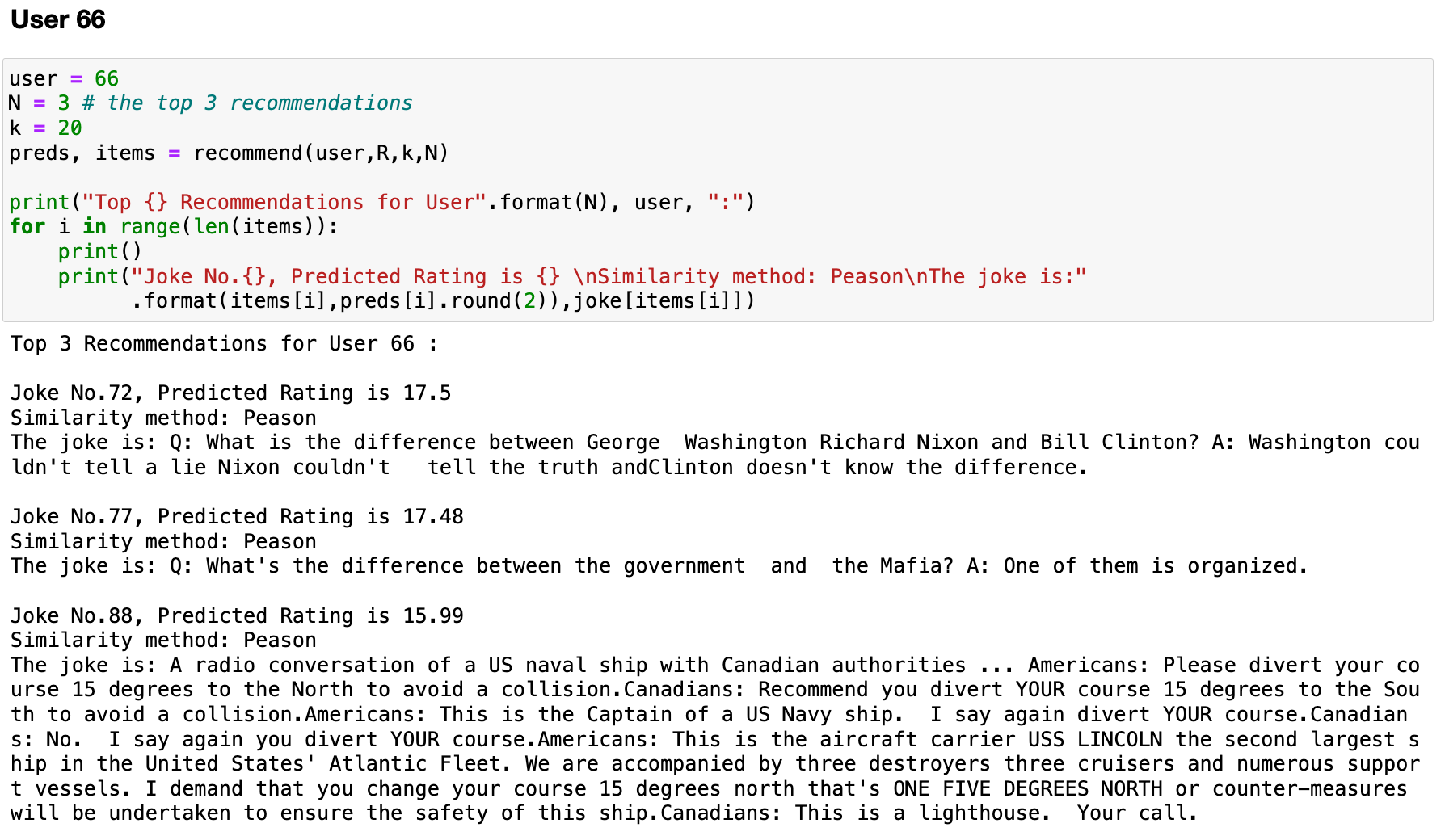
Methodology
and Approach
📝 Summary of Recommender System Built
Recommender Type | Technical Approach | Application Scenario |
---|---|---|
Popularity-Based Recommender | Based on global item popularity | Recommending trending products or content during cold-start situations |
Non-personalized Item Similarity Recommender | Based on item-item similarity using feature vectors | “Customers also viewed” modules in e-commerce platforms like Amazon, Etsy |
Linear Regression Recommender | Predicting ratings using user and item features through supervised learning (Ridge Regression) | Predicting product or content quality for rating prediction models |
User-Based Collaborative Filtering | Finding similar users based on co-rated items (Pearson correlation) | Friend or follower recommendations in social networks like Facebook |
Item-Based Collaborative Filtering | Finding similar items based on user ratings (Cosine similarity) | Product recommendation systems such as Amazon |
Personalized Content-Based Recommender | Building individual user profiles based on previously liked items (TF-IDF aggregation) | Personalized content feeds on Netflix, Spotify, YouTube |
Non-personalized Content-Based Recommender | Recommending most similar items to a given item using content features | Similar product recommendations on item pages (Amazon, Etsy) |
1. Non-personalized Recommendation
- Popularity-Based Recommender
Predicted ratings based on global average item ratings; useful as a baseline or cold-start fallback. - Non-personalized Item Similarity Recommender
Returned Top-N most similar items based on item-item cosine similarity, independent of user preferences.
2. Feature-Based Regression Models
- Linear Regression Recommender Modeled rating prediction as a supervised learning problem using user and item features. Ridge regularization was applied to mitigate overfitting and tested across different alpha values.
3. Collaborative Filtering
- User-Based Collaborative Filtering
Computed user-user similarity matrix via Pearson correlation. Predicted ratings by aggregating ratings from k-nearest neighbors. - Item-Based Collaborative Filtering
Built item-item similarity matrix using cosine similarity on user rating vectors. Predicted ratings based on weighted contributions from similar items rated by the user. - Model Evaluation
RMSE and MAE were computed to evaluate performance across different k values.
4. Content-Based Recommendation
- Personalized Content-Based Recommender
Built user profiles by aggregating features of liked items based on TF-IDF representations. Recommendations generated by cosine similarity between user profiles and unseen items. - Non-personalized Content-Based Recommender
Recommended Top-N most similar items to a given target item using item feature similarity. - Model Evaluation
Precision@k and Recall@k were computed and plotted across different k values to evaluate personalized recommendations.
Results and Impact
- Successfully implemented 7 different types of recommender models from scratch.
- Demonstrated deep understanding of recommendation system concepts such as:
- Similarity metrics (cosine, Pearson)
- Neighborhood-based prediction (kNN)
- User profiling and item vectorization
- Achieved solid RMSE/MAE scores on collaborative filtering models.
- Achieved high precision/recall scores on personalized content-based recommendations.
- Built a clear, reproducible, and extensible codebase for future expansion.
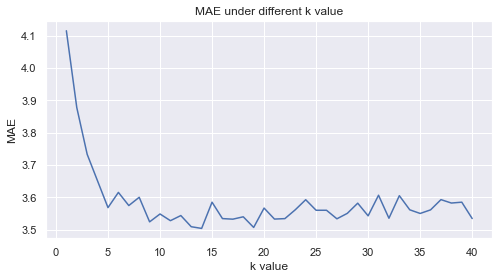
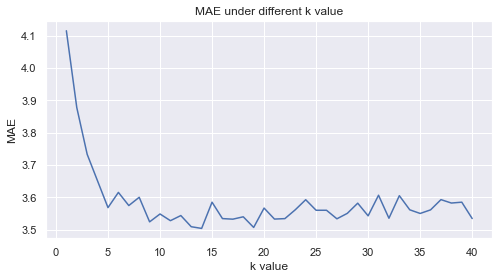
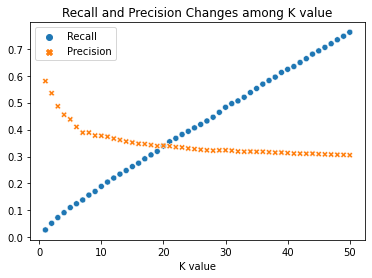
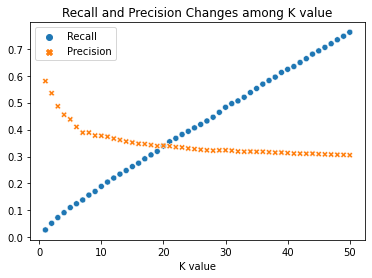
Access Full Details and Files
For full project details, source files, and additional insights, visit the GitHub repository.