Credit Card Churn Prediction
– Powered by GridMaster
Churn Prediction · Automated Hyperparameter Tuning· Customer Retention
Overview
This project presents a complete end-to-end pipeline for predicting customer churn in the credit card industry. It combines robust data science practices with a powerful custom tool I built – GridMaster.
It includes everything from data cleaning and exploratory analysis to multi-model grid search, model comparison, and actionable insights. The third notebook also serves as a showcase for GridMaster’s advanced capabilities, positioning this portfolio piece as both a project delivery and a product demonstration.
Technology Stack
- Programming Language:
python - Libraries:
scikit-learn, matplotlib, seaborn, XGBoost, lightGBM, CatBoost - Self-Developed Library:
GirdMaster - Development Environment:
VS Code, Atom, Jupyter Notebook - Version Control:
Git & GitHub
Methodology
and Approach
- Data Cleaning: Removed redundant columns, transformed categorical variables, and re-encoded the binary target
Attrition_Flag
to 0/1. - Exploratory Data Analysis: Visualized feature distributions, churn patterns, and feature correlations; summarized insights from age, transaction behavior, income level, and card category.
- Baseline Modeling: Built a logistic regression classifier to establish a benchmark performance.
- Advanced Model Development:
- Used GridMaster to automate coarse-to-fine grid search across Logistic Regression, Random Forest, and XGBoost models.
- Specified scoring priority as recall, reflecting the business need to minimize false negatives in churn detection.
- Executed multi-stage search with custom parameter grids and 5-fold cross-validation.
- Visualized parameter tuning curves and feature importances.
What is GridMaster
GridMaster is a mature, custom-developed Python package designed to extend and generalize the capabilities of tools like GridSearchCV
.
Unlike GridSearchCV
which handles one model at a time, GridMaster supports:
- Multi-model coordination: Tune multiple classifiers in a single pipeline
- Coarse-to-fine hyperparameter search: Efficiently move from wide exploration to focused refinement
- Custom scoring metrics: Optimize for recall, F1, ROC-AUC, etc.
- Visual analysis: Plot parameter-performance curves and feature importance
- Single-call execution: Run the full pipeline with just a few lines of code
The package is open-source, production-ready, and suitable for both quick experimentation and enterprise-scale ML workflows.
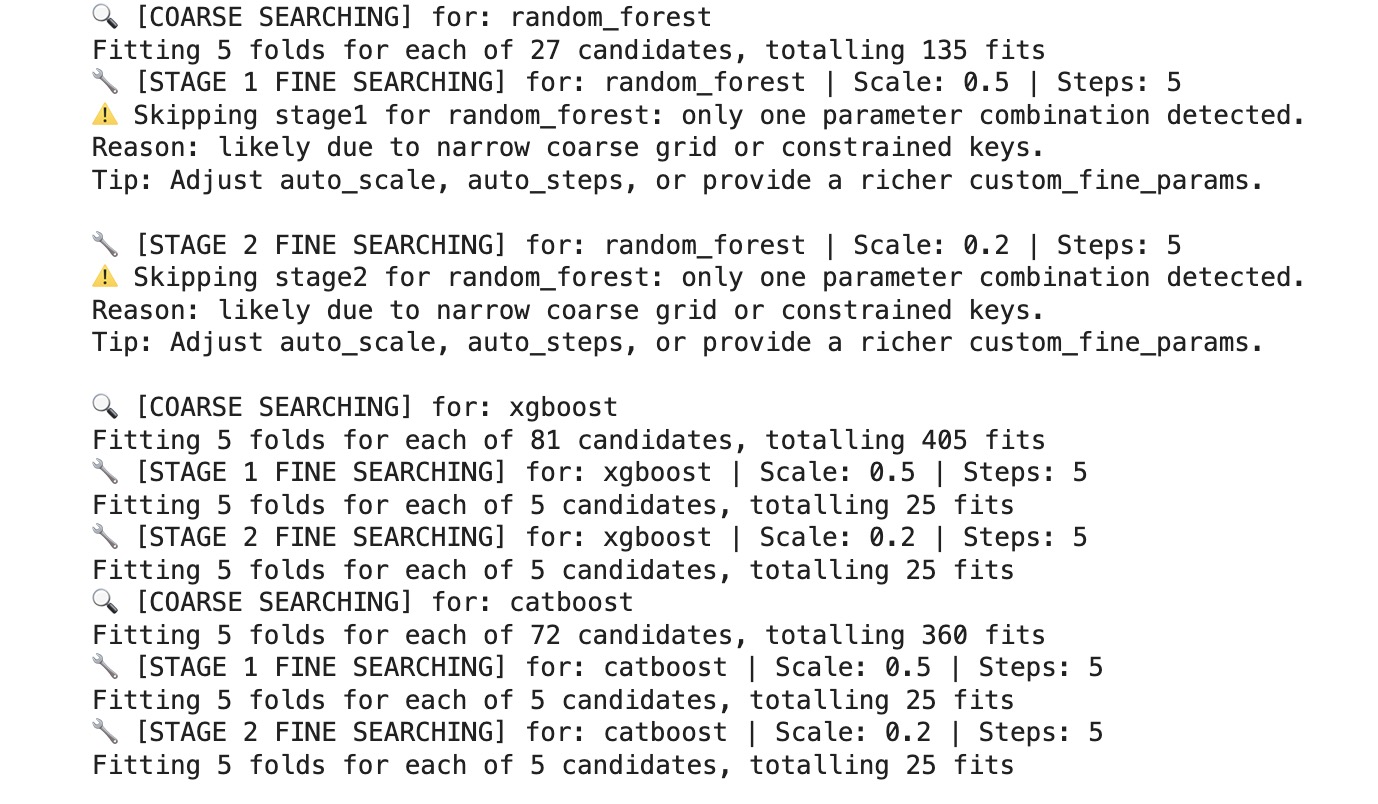
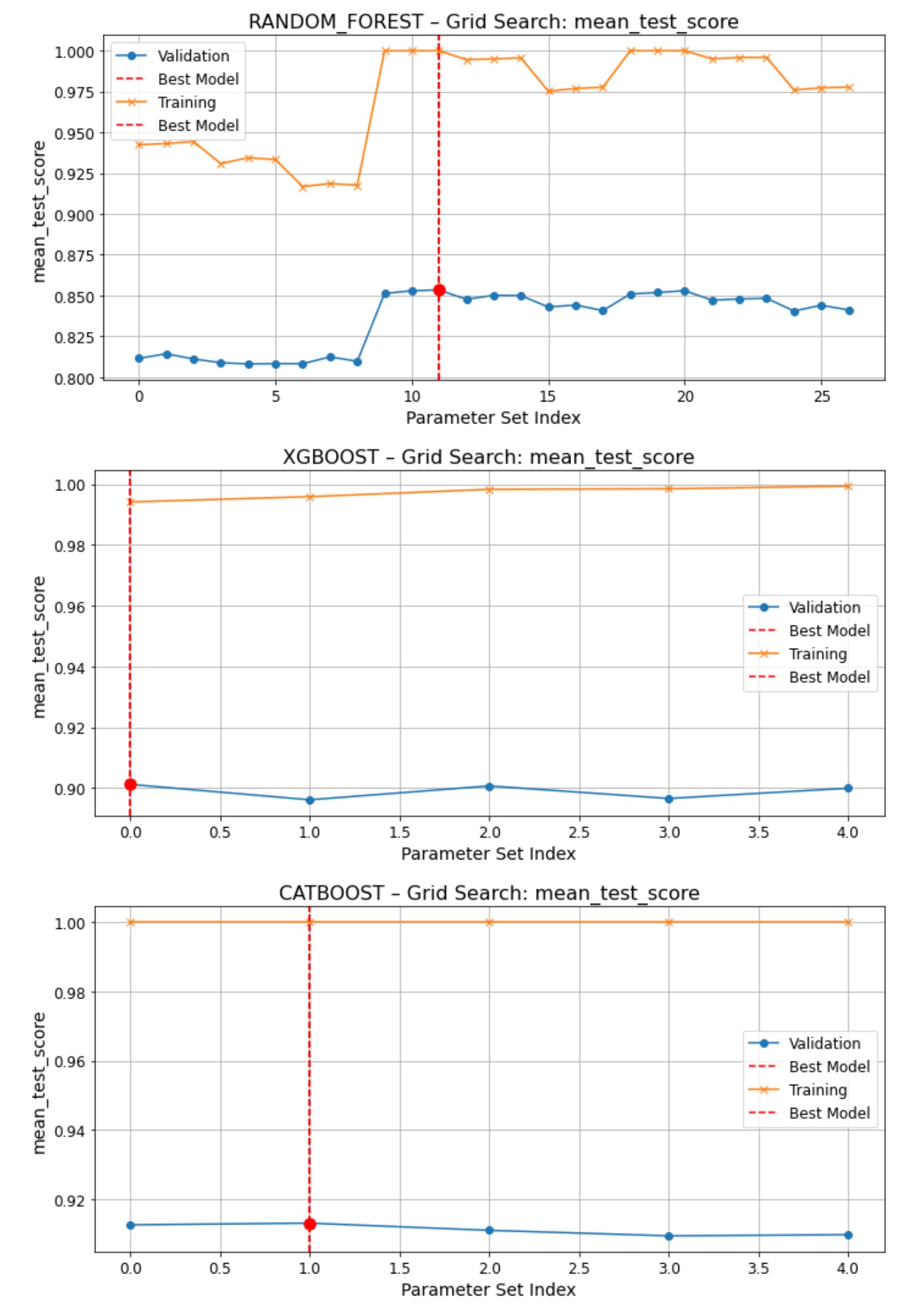
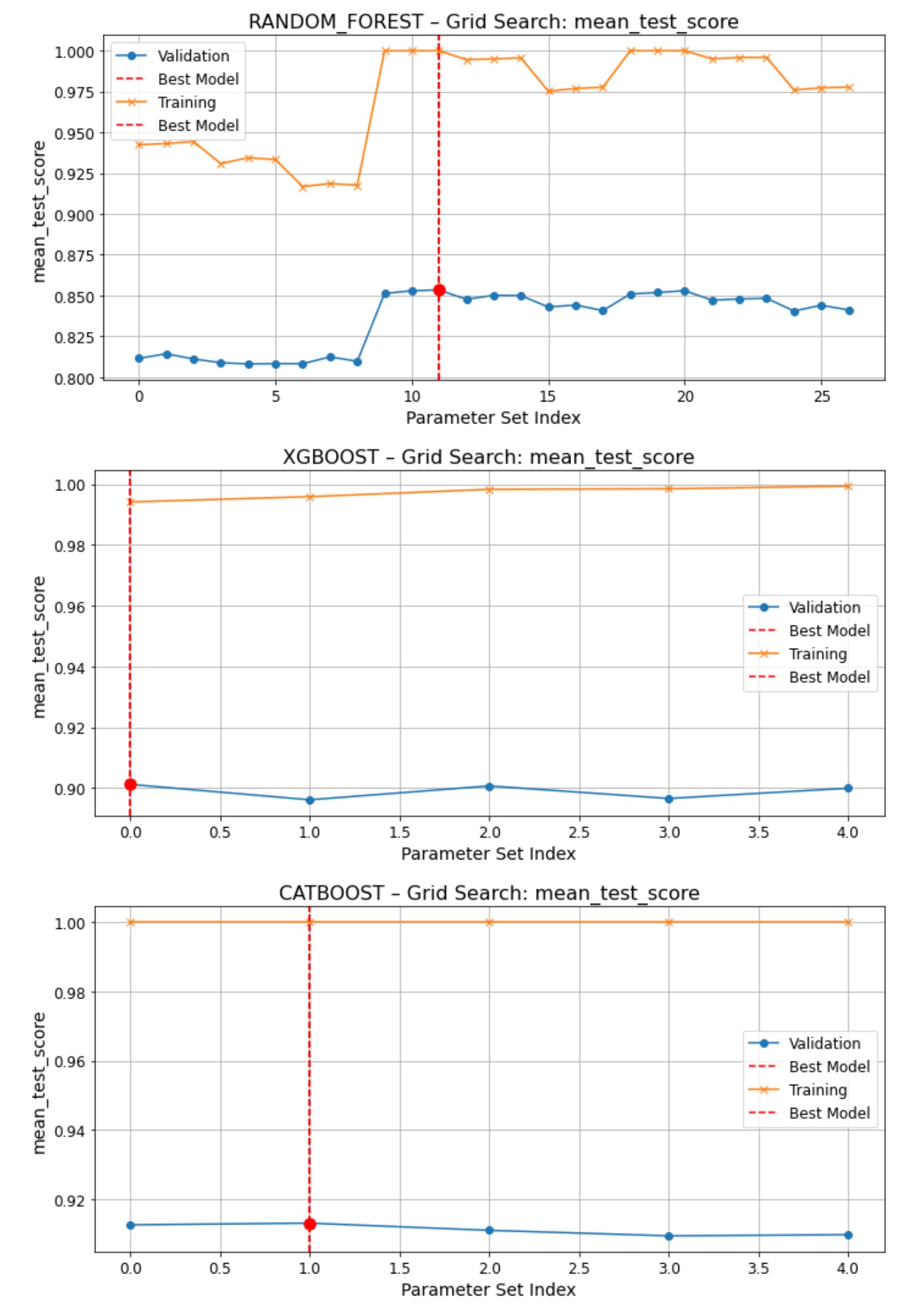
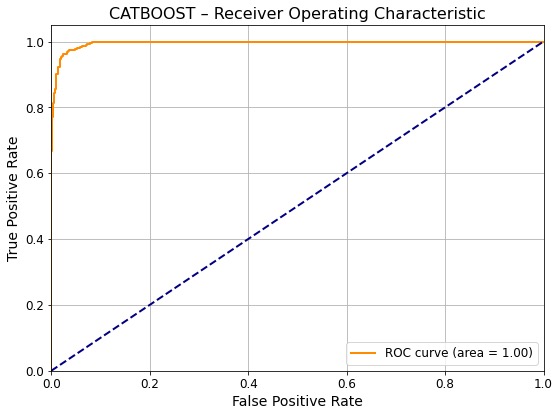
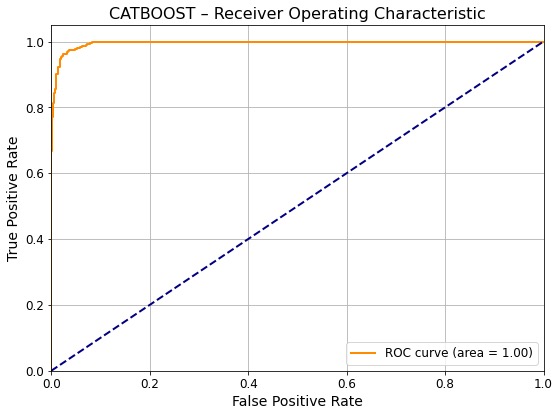
Why GridMaster
over
GridSearchCV?
While GridSearchCV
from scikit-learn is a powerful tool for tuning hyperparameters, it has several limitations when scaling to complex workflows. GridMaster was developed to address those limitations and provide a more flexible, scalable, and insightful grid search experience.
Feature | GridSearchCV | GridMaster |
---|---|---|
Multi-model coordination | ❌ One model per run | ✅ Tune and compare multiple models in one run |
Multi-stage tuning (coarse/fine) | ❌ Manual splitting | ✅ Built-in pipeline with smart defaults |
Visualization of search results | ❌ Not included | ✅ Parameter-performance plots, feature importance |
Default scoring flexibility | ✅ Yes | ✅ Fully configurable and per-model optional |
Silent training output | ❌ No suppression | ✅ Optional suppression for cleaner logs |
Quickstart usability | ⚠️ Requires boilerplate | ✅ 3-line setup for end-to-end search |
Designed for notebook analysis | ⚠️ Limited interactivity | ✅ Built for visual exploration + summary tables |
GridMaster isn’t a replacement for GridSearchCV — it’s a natural evolution of it, ideal for practitioners who want both power and usability in model optimization workflows.
Results & Insights
- Best model for minimizing false negatives (capture as many actual churned customers as possible):
XGBoost with a recall score of 0.8794 - Optimal parameters:
{'clf__learning_rate': 0.1824, 'clf__max_depth': 3, 'clf__n_estimators': 200}
- Best Model for Balanced Performance (Less bothering non-churning users):
Catboost with an f1 score of 0.9131 - Optimal parameters:
{'clf__learning_rate': 0.07667317, 'clf__depth': 4, 'clf__iterations': 500, 'clf__l2_leaf_reg': 1}
- Key Features Identified:
Total_Trans_Ct
,Total_Trans_Amt
, andContacts_Count_12_mon
emerged as the strongest indicators of churn. - Business Takeaway: GridMaster streamlined the experimentation process while maintaining performance rigor. High recall ensures fewer missed churn cases, supporting timely customer retention strategies.
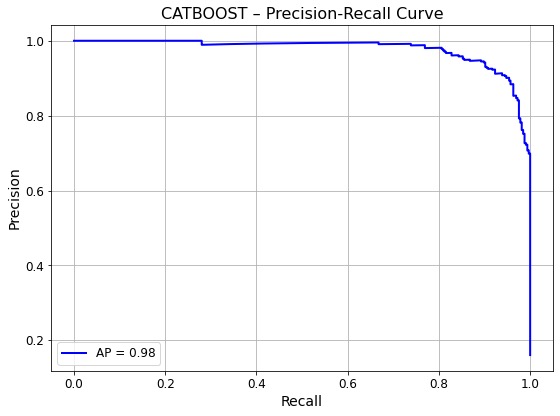
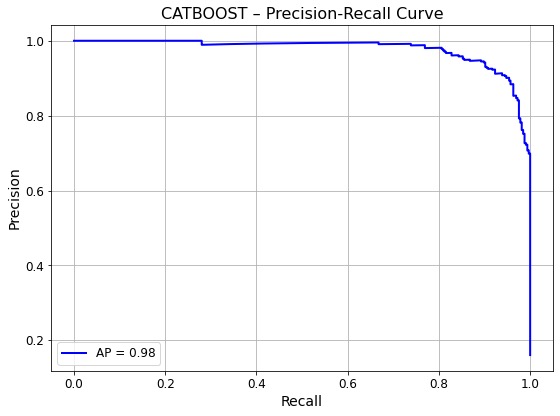
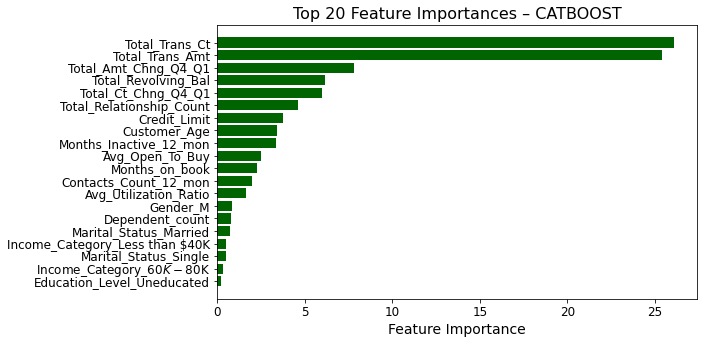
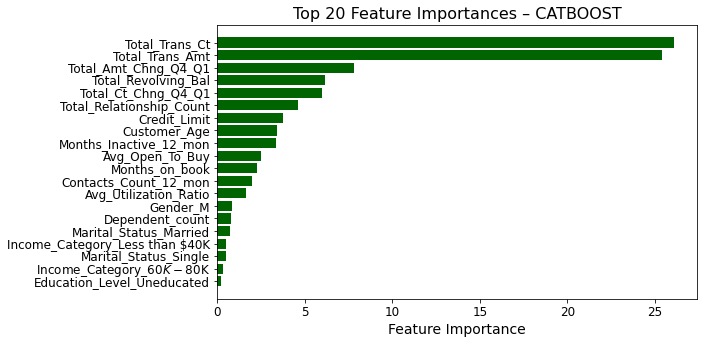
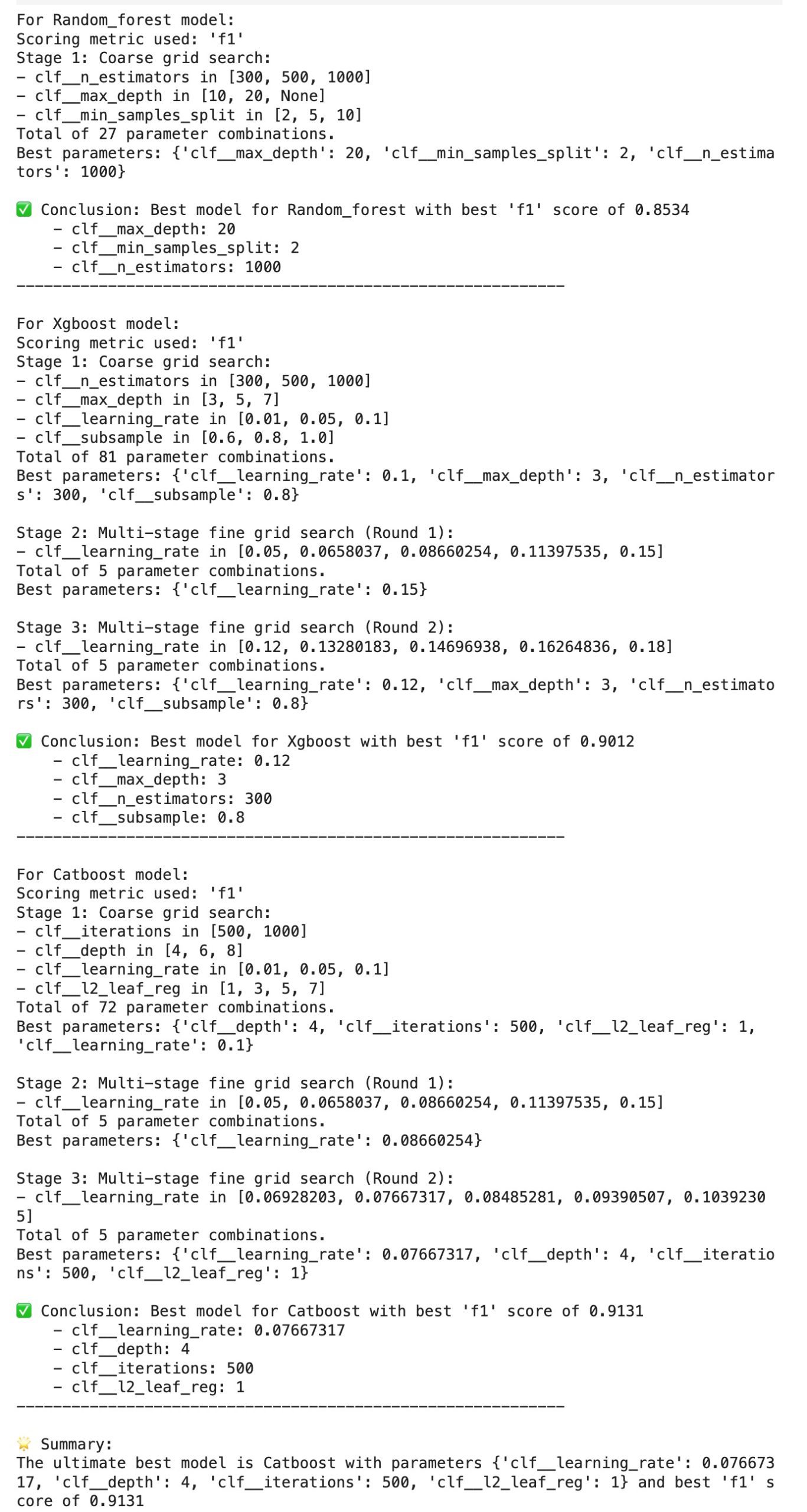
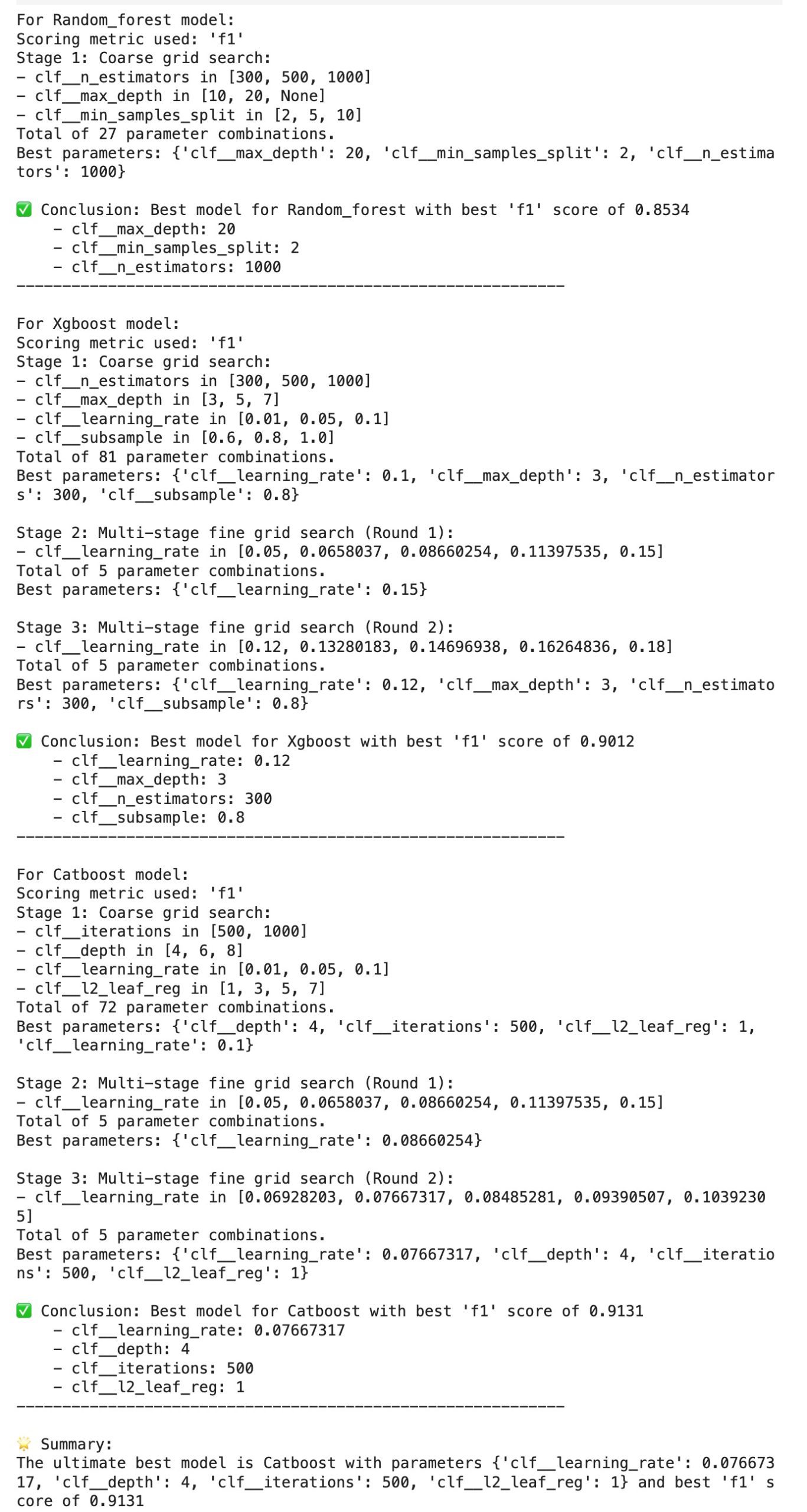
Access Full Details and Files
For full project details, source files, and additional insights, visit the GitHub repository.