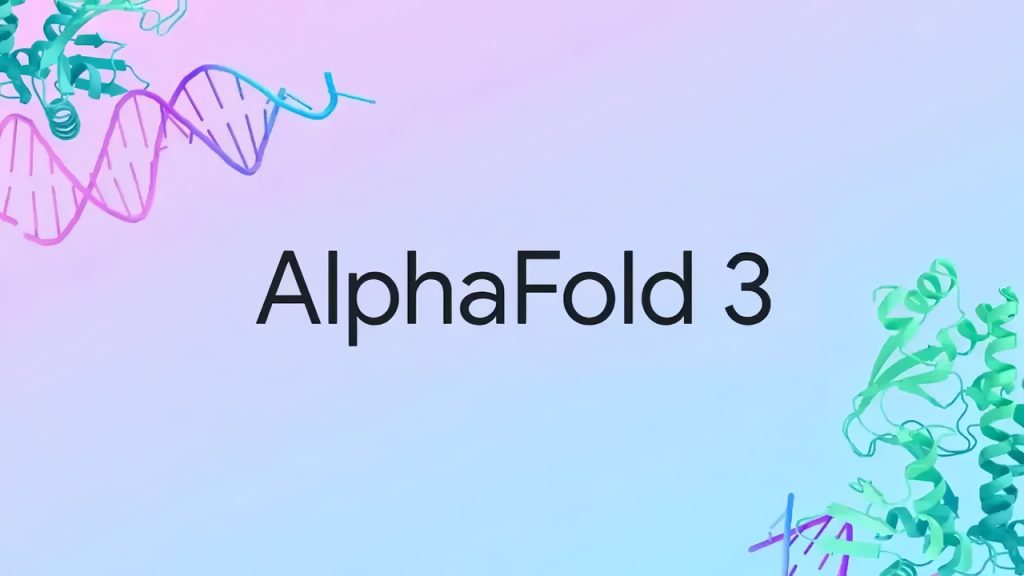
On October 9, 2024, the Royal Swedish Academy of Sciences decided to award half of the 2024 Nobel Prize in Chemistry to Demis Hassabis and John Jumper for their development of AlphaFold2 in 2020, a model capable of predicting the structure of almost all 200 million proteins discovered by researchers. Here is the official scientific background: They have revealed proteins’ secrets through computing and artificial intelligence.


The introduction of AlphaFold2 triggered a revolution in protein structure modeling and interaction prediction, giving rise to numerous applications in protein modeling and design. More than three years later, on May 8, 2024, Google DeepMind released the next-generation AlphaFold 3 (AF3), which brought significant updates. Today, we will briefly explore AlphaFold 3 through the article published by Google DeepMind in Nature.
AlphaFold 3 (AF3) is the latest deep learning model developed by DeepMind for predicting the structure of biomolecular complexes, including proteins, nucleic acids, small molecules, ions, and modified residues. Unlike previous tools that specialized in predicting specific interaction types, AF3 achieves highly accurate modeling across the biomolecular space within a single framework.
Key Features
1. Protein Structure Prediction:
AF3 can predict the structures of various proteins, including monomers, multimers, and large proteins with thousands of residues.
2. Protein Interaction Prediction:
Notably, the accuracy of predicting antibody-protein interactions has significantly improved.
3. Protein-Ligand Interaction Prediction:
AF3 can accurately predict protein-ligand interactions, including small molecules, ions, and modified residues. It can handle a broad range of chemical space without needing separate protein structure prediction and ligand docking processes.
4. Protein-Nucleic Acid Interaction Prediction:
AF3 is capable of high-precision predictions of protein-nucleic acid complexes, including large structures with thousands of residues.
5. Covalent Modification Prediction:
AF3 can accurately predict covalent modifications, including bonded ligands, glycosylation, and modifications involving any polymer residue (protein, RNA, or DNA).
Application Areas
• Understanding Cellular Function: Accurate structural models of biomolecular complexes are crucial for understanding cellular functions.
• Rational Therapeutic Design: It helps design small molecules that bind to specific biomolecular targets.
• Protein Engineering and Design: Useful for designing new proteins with specific functions.
• Basic Biological Research: Assists researchers in understanding biomolecule interaction mechanisms.
Model Architecture
AF3 retains a similar overall structure to AF2, featuring a large trunk network that evolves pairwise representations of chemical complexes and a structure module that uses the pairwise representation to generate atomic positions. Combining simplified MSA processing, diffusion-based generative structure prediction and strong pairwise representations enables AF3 to achieve high accuracy in predicting a wide range of biomolecular complexes.


Pairformer Module
AlphaFold 3 replaces AlphaFold 2’s Evoformer module with the new Pairformer module. Pairformer focuses on handling pairwise representations, working only with paired chemical complex representations and discarding the MSA (multiple sequence alignment) information. This allows AlphaFold 3 to capture molecular interactions efficiently. Unlike AlphaFold 2, AlphaFold 3 substantially reduces its reliance on MSA, retaining a simple MSA embedding block while relying primarily on pairwise representations for structure prediction.
Diffusion Module
AlphaFold 3 introduces a diffusion module that directly processes atomic coordinates, unlike AlphaFold 2, which relies on amino acid-specific frames and side-chain torsion angles. The diffusion model generates molecular structures by denoising atomic coordinates, allowing the model to learn both local and global structures at different noise levels. The multiscale nature of the diffusion process allows AlphaFold 3 to eliminate the complex torsion angle and chemical bond constraints present in AlphaFold 2, making it better suited for arbitrary chemical structures.
New Training Mechanism
AlphaFold 3 uses a generative diffusion model training mechanism. During training, the model gradually generates complete structures through denoising, allowing AlphaFold 3 to generate multiple candidate structures for each molecule and select the most accurate one. The likelihood of the model generating biologically irrelevant structures is reduced by applying cross-distillation training, which uses AlphaFold-Multimer 2.3’s structures for auxiliary training.
Training Process
Initial Training and Two Subsequent Fine-Tuning Phases
Phase 1: Initial Training
During the initial training phase, the model uses a crop size of 384 tokens. Each optimizer step processes a mini-batch of 256 input data samples, generating 256 × 48 = 12,288 diffusion samples.
In this phase, the model quickly learns to predict local structures, with all intrachain metrics rising rapidly and reaching 97% of maximum performance within the first 20,000 training steps. However, learning global structures, such as the LDDT metric for protein-protein interfaces, takes longer, reaching 97% after 60,000 steps.
Researchers increased or decreased the sampling probability for the corresponding training sets to address overfitting in certain capabilities and applied early stopping strategies.
Phase 2 and 3: Fine-Tuning
The model underwent two subsequent fine-tuning stages with crop sizes of 640 and 768 tokens, respectively. Each fine-tuning step uses mini-batches of 256 input data samples, reducing the number of diffusion samples to 256 × 32 = 8,192. These fine-tuning stages improved the model’s performance on all metrics, particularly in protein-protein interfaces.
Training data was cut off as of September 30, 2021, ensuring no structural data released after that date was used during training. To address hallucination issues, the researchers enriched the training data with structures predicted by AlphaFold-Multimer (v.2.3).


Dealing with Hallucination
As mentioned earlier, due to the generative nature of the diffusion model, AF3 is prone to hallucination issues, where the model may generate plausible-looking structures in unstructured regions of biomolecular complexes.
AF3 uses a cross-distillation method to tackle the hallucination problem. It enriches the training data with structures predicted by AlphaFold-Multimer (v.2.3). In these structures, unstructured regions are typically represented by long loops rather than compact structures, and training on these helps AF3 mimic this behavior, thereby reducing hallucination.
Additionally, AF3 has developed confidence measures that predict atom-level and pairwise errors in the final structures, helping users identify potential hallucination regions.
Confidence and Accuracy
The article comprehensively evaluates the accuracy of the AF3 model across various biomolecular complex types:


• Protein-Ligand Interaction: AF3 was evaluated on the PoseBusters benchmark set (comprising 428 protein-ligand structures released to the PDB in 2021 or later). Without using any structural inputs, AF3 greatly outperformed classical docking tools like Vina (P = 2.27 × 10⁻¹³) and all other true blind docking tools like RoseTTAFold All-Atom (P = 4.45 × 10⁻²⁵).
• Protein-Nucleic Acid Interaction: AF3 achieves higher accuracy than RoseTTAFold2NA in predicting protein-nucleic acid complexes and RNA structures. AF3 can predict structures with thousands of residues.
• Covalent Modifications: AF3 successfully predicts 75.8% of bonded ligands (n = 66 clusters) and 56.9% success rate for single-residue glycosylation (n = 28 clusters) on high-quality experimental data.
• Protein Complexes: AF3 shows improved success rates (DockQ > 0.23) in predicting protein-protein interactions compared to AlphaFold-Multimer (v.2.3) (P = 1.8 × 10⁻¹⁸), with notable improvements in antibody-protein interaction prediction (P = 6.5 × 10⁻⁵). AF3 also significantly improves protein monomer LDDT (P = 1.7 × 10⁻³⁴).
Limitations
- Stereochemistry Violations: The model’s predictions may occasionally not respect chirality or produce overlapping (clashing) atoms.
- Hallucination: AF3 may generate spurious structures in unstructured regions, although these areas are typically marked with low confidence.
- Dynamical Limitations: AF3 primarily predicts static structures and does not fully simulate the dynamic behavior of biomolecular systems in solution.
- Limited Conformational Coverage: AF3 may not predict all possible conformational states for certain targets.
- Lower Accuracy for Certain Targets: To achieve high accuracy, some targets may require generating a large number of predictions and ranking them, increasing computational costs.
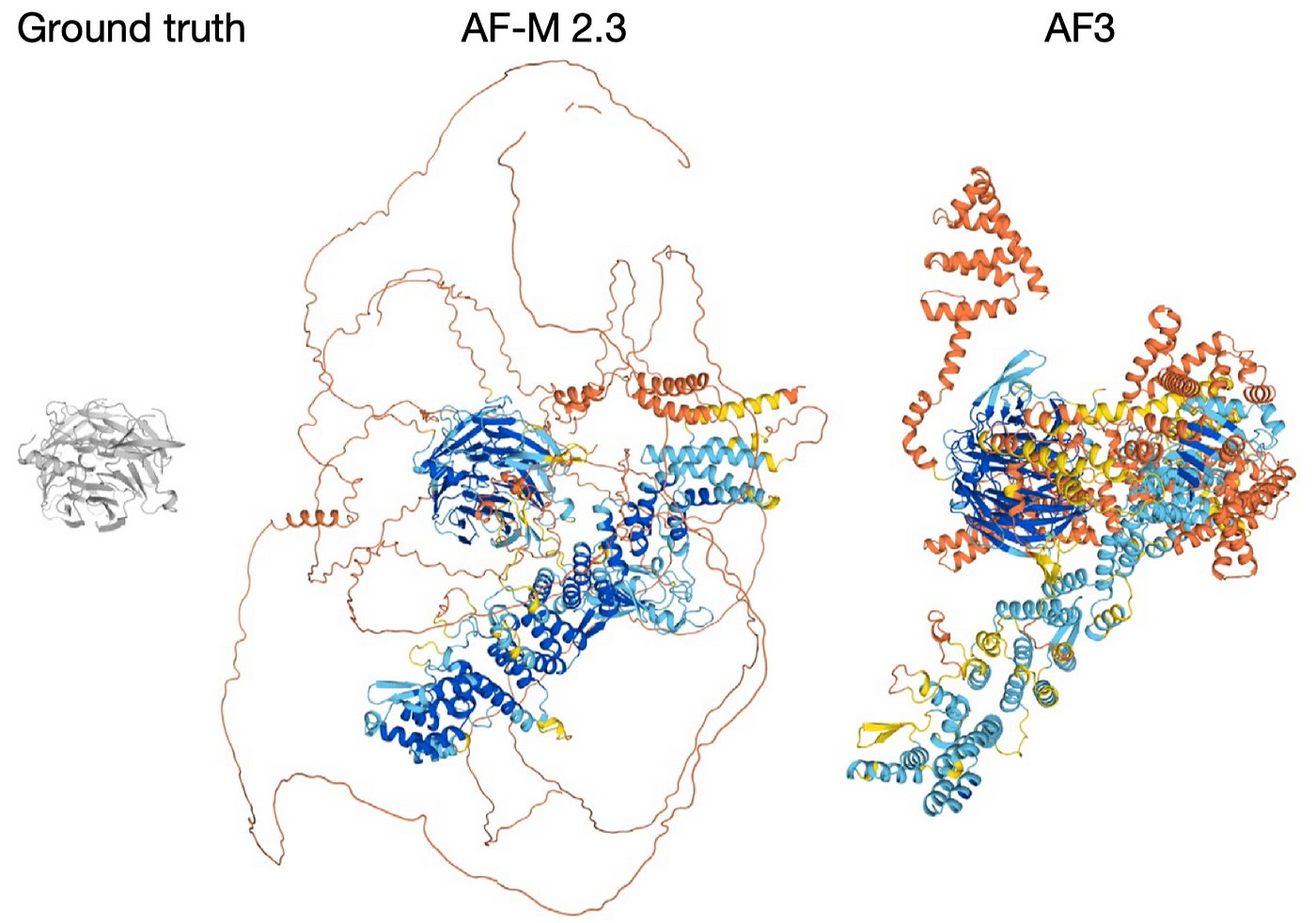
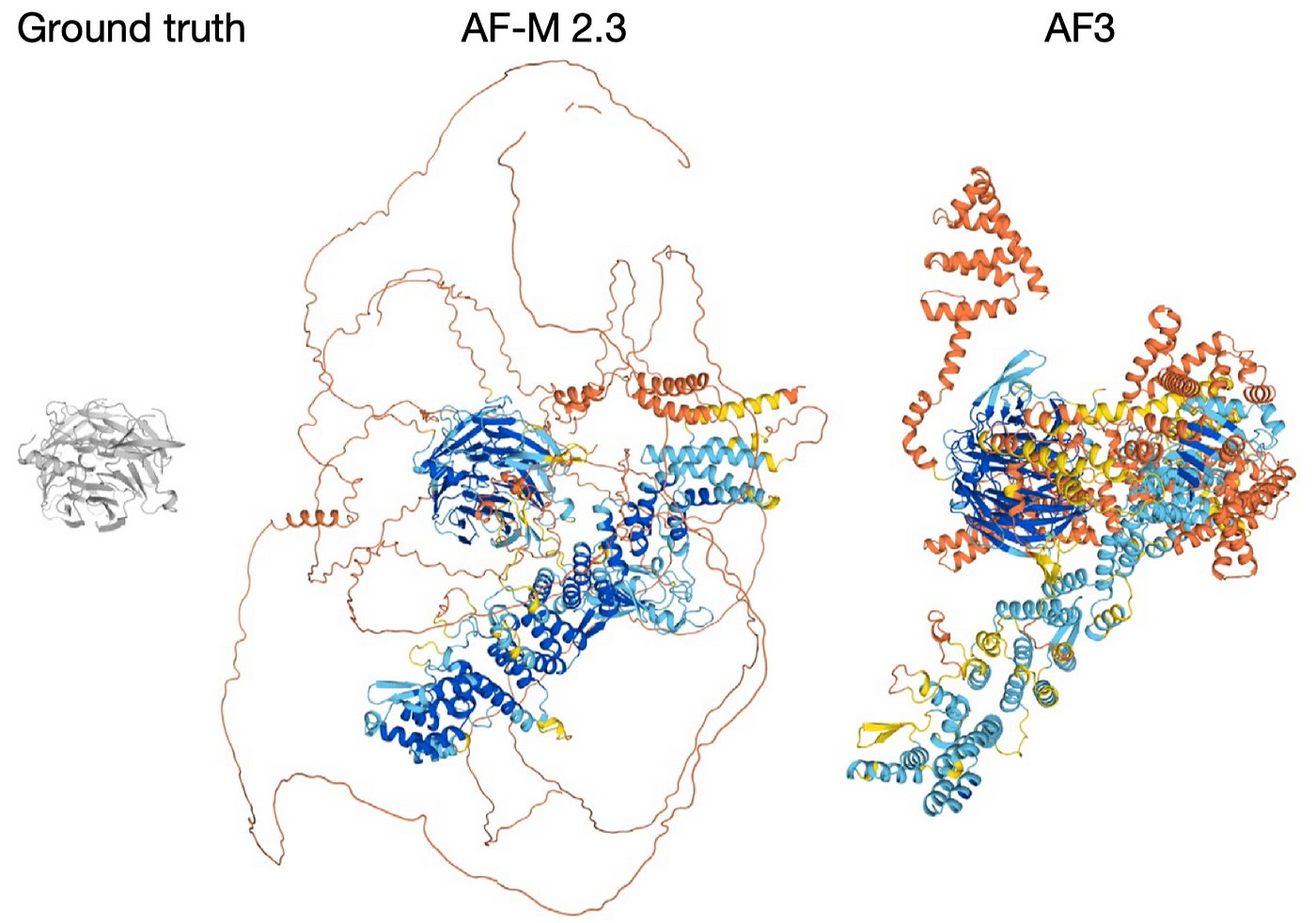
Summary of Advantages
Compared to earlier protein structure prediction tools and previous versions of AlphaFold, AF3 offers the following key advantages:
1. Expanded Biomolecular Prediction Capabilities: Unlike previous versions, which primarily focused on protein structure prediction, AF3 can predict the structures of various biomolecular complexes, including proteins, nucleic acids, small molecules, ions, and modified residues. This expanded capability makes AF3 a more versatile tool applicable to various biological research areas.
2. Improved Prediction Accuracy: AF3 demonstrates higher prediction accuracy in several areas:
• Protein-Ligand Interaction: AF3 outperforms state-of-the-art docking tools (such as Vina), even without structural inputs.
• Protein-Nucleic Acid Interaction: AF3 surpasses nucleic-acid-specific tools like RoseTTAFold2NA in predicting protein-nucleic acid complexes.
• Antibody-Antigen Interaction: AF3 shows marked improvements in antibody-antigen prediction accuracy compared to AlphaFold-Multimer v.2.3.
• Protein Monomers and Complexes: AF3 improves prediction accuracy for protein monomers and complexes compared to AlphaFold-Multimer v.2.3.
3. Unified Deep Learning Framework: AF3 uses a single deep learning framework to predict all biomolecular interactions, eliminating the need to develop specialized models for each interaction type. This unified framework simplifies model development and usage while improving generalization.
4. Improved Confidence Measures: AF3 employs a new diffusion “rollout” method for developing confidence measures, allowing it to predict atom-level and pairwise errors in the final structure. These confidence measures help users assess the reliability of the predictions.
Improvements Over AlphaFold 2 (AF2)
AF3 brings several improvements over AF2, including:
• Updated Architecture: AF3 uses a diffusion-based architecture that directly predicts atomic coordinates, eliminating the need for rotational frames or equivariant processing.
• Simplified MSA Processing: AF3 replaces AF2’s “evoformer” with a simpler and more efficient “pairformer” module, reducing its reliance on multiple sequence alignments (MSA).
• Enhanced Generalization: AF3 can predict complex structures involving almost all molecular types found in the Protein Data Bank (PDB), including ligands, ions, nucleic acids, and modified residues.
• Higher Prediction Accuracy: AF3 outperforms AF2 and other specialized tools in several areas, including protein structure, protein-protein interactions, protein-ligand interactions, protein-nucleic acid interactions, and antibody-antigen predictions.
Future Directions
• Improving Accuracy for All Interaction Types: Further improvements in model architecture, training procedures, and data will continue to enhance AF3’s accuracy in predicting all biomolecular complexes.
• Enhancing Modeling of Biomolecular Dynamics: New methods will be developed to simulate the dynamic behavior of biomolecules in solution, such as conformational changes and flexibility.
- Expanding to Larger Biomolecular Systems: AF3’s capabilities will be expanded to predict the structures of more complex biomolecular systems, such as viruses and organelles.