Over the past year, the explosion of generative AI has flooded the world with AI-generated content. As we move forward, training future models on this machine-generated data seems inevitable. A recent study explores the potential issues when large language models are trained using data generated by other models.
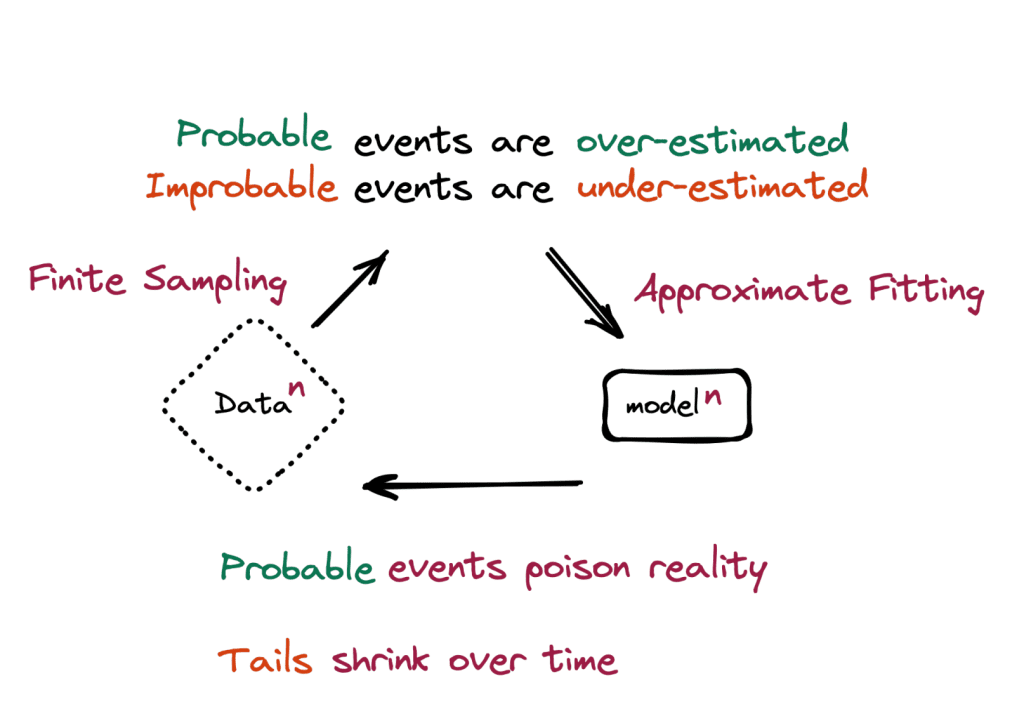
The researchers found that as training progresses across generations, models begin to diverge from the true data distribution, a phenomenon known as Model Collapse. This leads to performance degradation, especially with low probability events.
This research underscores the critical need for careful data selection and validation in training AI models. To avoid model collapse, we must prioritize the use of real, human-generated data. Looking ahead, the industry should consider implementing strategies like content tagging and human feedback to maintain data quality. Establishing clear standards and guidelines will be essential to ensure these practices are effectively adopted and implemented.
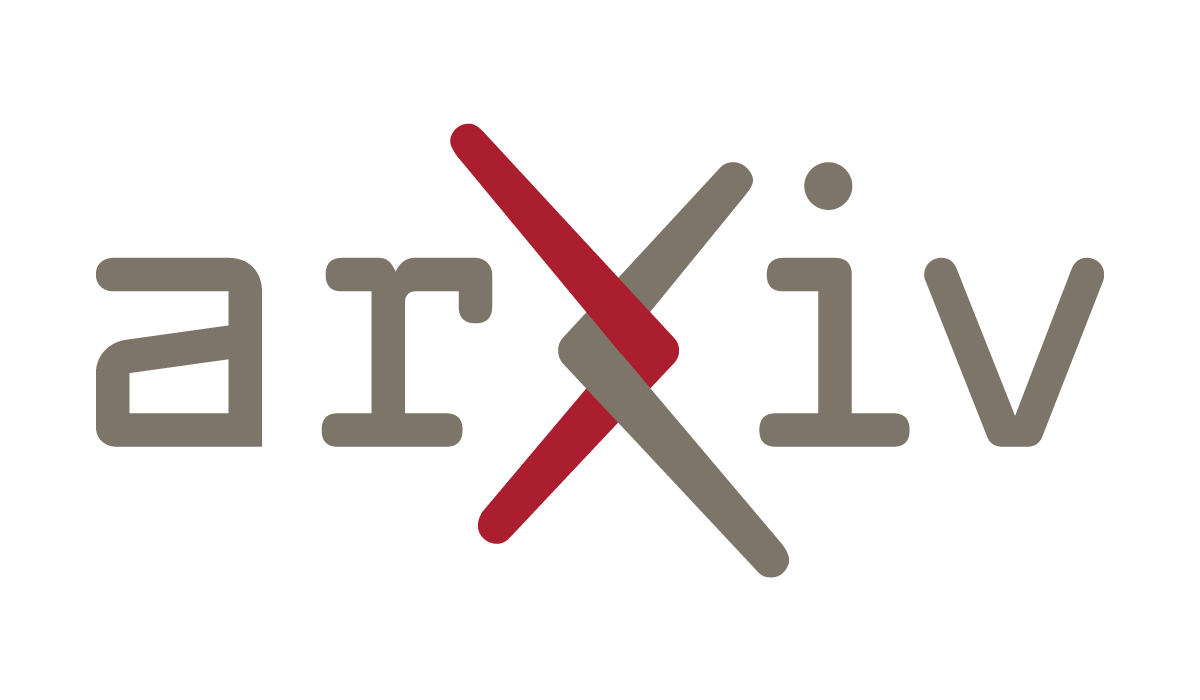
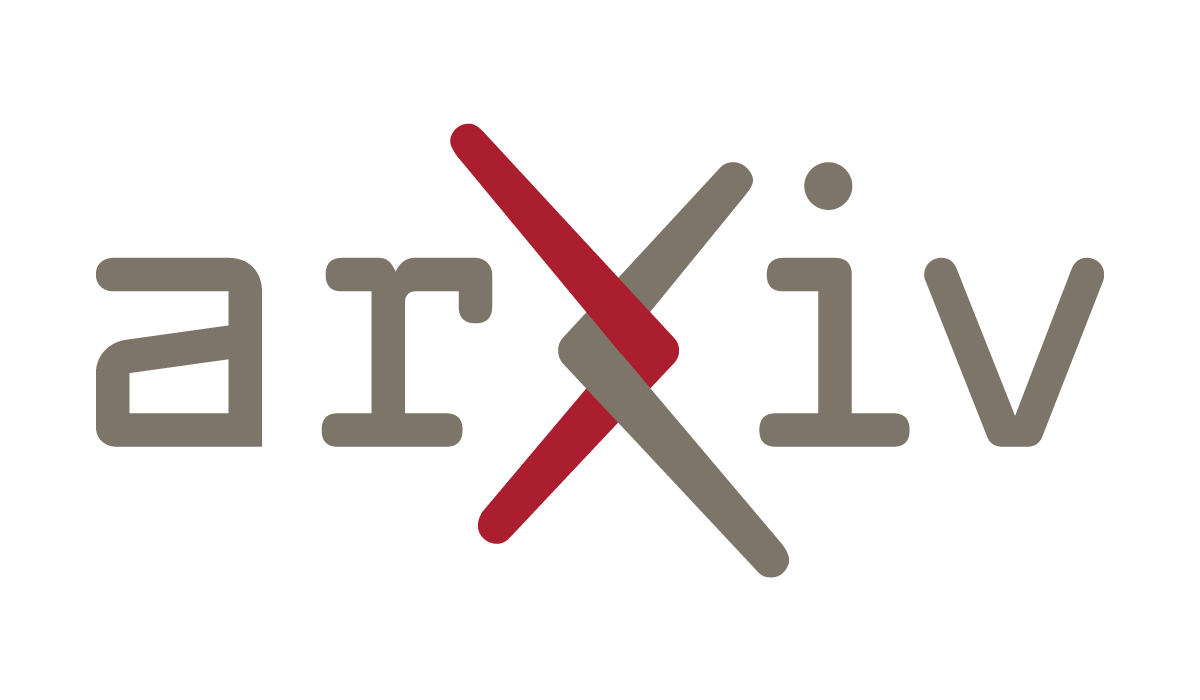